|
|
人工智能对烧伤创面的辅助治疗 | Burns & Trauma |
|
论文标题:Burn image segmentation based on Mask Regions with Convolutional Neural Network deep learning framework: more accurate and more convenient
期刊:Burns & Trauma
作者:Chong Jiao, Kehua Su, Weiguo Xie and Ziqing Ye
发表时间:2019/02/28
数字识别码:10.1186/s41038-018-0137-9
原文链接:https://burnstrauma.biomedcentral.com/articles/10.1186/s41038-018-0137-9?utm_source=other&utm_
medium=other&utm_content=null&utm_campaign=BSCN_2_WX_BurnsTrauma_Arti_Scinet
微信链接:https://mp.weixin.qq.com/s/9UQoujBQ0PwHivtn66iuag
从2016年AlphaGo击败世界围棋卫冕冠军李世石开始,人工智能技术就如潮水般蔓延至全球各地。自动驾驶、智能机器人、机器视觉、人脸识别等都在运用人工智能技术。近些年,随着医院设备的逐步完善,人工智能技术在医学研究中也得到了进一步的发展。
Burns & Trauma精选文章
Research
Burn image segmentation based on Mask Regions with Convolutional Neural Network deep learning framework: more accurate and more convenient
引用格式: Jiao C, Su K, Xie W, Ye Z. Burn image segmentation based on Mask Regions with Convolutional Neural Network deep learning framework: more accurate and more convenient. Burns Trauma. 2019 7:6
人工智能技术用于烧烫伤
烧烫伤是全球第六位伤害死因,据统计,中国每年的烧伤发生率超过1%,造成了沉重的社会负担、经济负担。及时、准确的烧伤程度评估,是为烧伤患者提供准确补液量,以及确定进一步治疗方案的关键前提,直接影响到烧伤患者的创面治疗和创后恢复,甚至关乎烧伤患者生命。一般认为,对于烧伤患者的治疗主要包括烧伤区域表面积计算和烧伤深度评估。传统的治疗手段是通过公式法或椭圆估算法估算出创面的面积,然后根据诊断经验对创面深度进行分类。这种方案往往带来巨大误差,错误判断甚至会给患者带来巨大伤害。近些年,随着计算机辅助设备的发展,基于数码相机、三维扫描仪的设备也开始应用于创面诊断。这些设备往往操作繁琐,无法解决复杂的临床使用环境,并且其昂贵价格也无法被某些中小型医院或诊所接受。据此,武汉大学计算机学院苏科华教授团队使用人工智能技术自动分割烧伤创面,仅通过手机对创面进行分析,避免繁琐的操作,对烧伤创面的治疗起到一定的辅助效果。
烧伤图像数据库
众所周知,训练一个优秀的深度学习框架往往需要大量带标签数据。因此,该团队与武汉三医院合作,在烧伤科门诊收集大量的病患创面图片,并通过微信的公众号平台将病患数据上传至服务器形成烧伤创面数据库。该团队通过研发的标注软件在专业医生的指导下,对烧伤创面进行标注,获得带标签数据集。图2展示数据收集和标注过程。
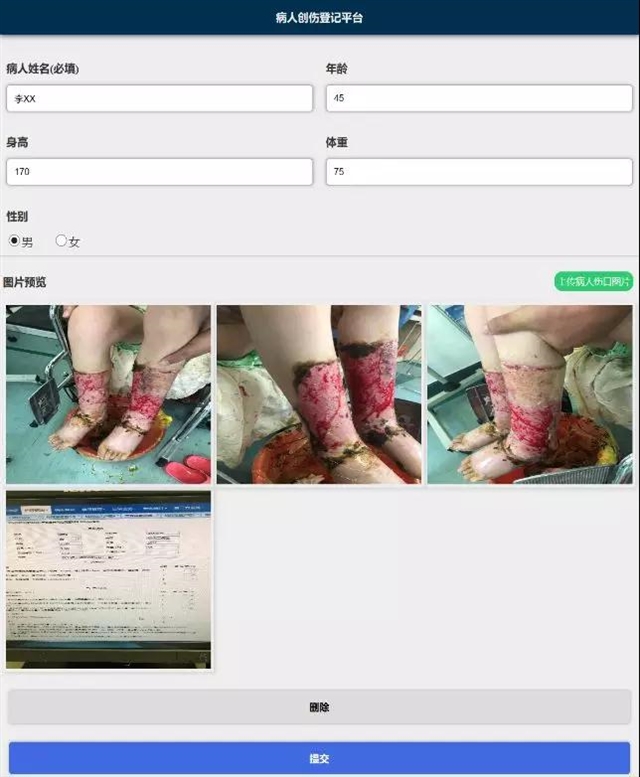
图2 数据收集和数据标注
基于Mask-RCNN的深度学习分割框架
2017年何凯明等人在Faster-RCNN的基础上提出了Mask-RCNN的实例分割框架。该框架采用残差网络作为深度卷积神经网络,通过区域候选网络来生成实例候选框,最后通过全卷积神经网络对实例进行分割,取得了优异的结果。武汉大学计算机学院苏科华教授团队在该框架的基础上,将多尺度卷积神经网络和区域候选网络结合起来,并改进了全卷积神经网络实例分割的分类函数,得到了基于烧伤的创面分割框架。整个框架如图3所示。
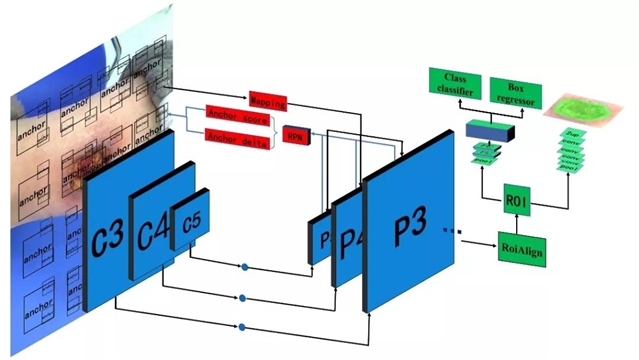
图3 烧伤创面分割框架
创面分割
一方面,烧伤创面分割框架具有较高的鲁棒性,对于不同类型、不同面积、不同深度的烧伤创面都表现出良好的分割结果。另一方面,烧伤创面分割框架移植性强,可在手机端复现,使用时仅需对病患的创面进行拍照即可,简单高效。图4是烧伤创面分割框架在不同创面下的分割结果展示。
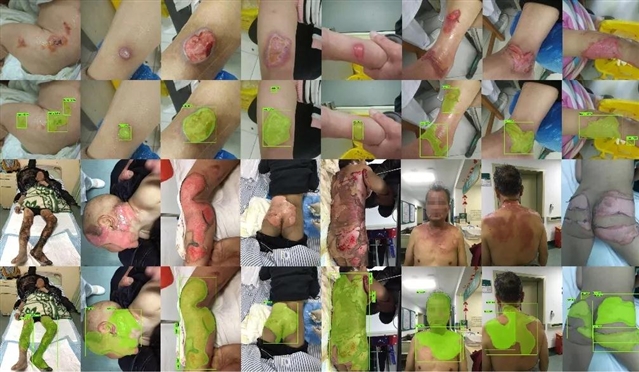
图 4烧伤创面分割框架分割结果
目前,该技术仅能分割创面来辅助创面的诊断,对于创面的深度评估,需要构建相应的创面深度标签数据集,进而训练创面深度评估框架。该团队表示,这也是下一步的研究重点。
Abstract
Background
Burns are life-threatening with high morbidity and mortality. Reliable diagnosis supported by accurate burn area and depth assessment is critical to the success of the treatment decision and, in some cases, can save the patient’s life. Current techniques such as straight-ruler method, aseptic film trimming method, and digital camera photography method are not repeatable and comparable, which lead to a great difference in the judgment of burn wounds and impede the establishment of the same evaluation criteria. Hence, in order to semi-automate the burn diagnosis process, reduce the impact of human error, and improve the accuracy of burn diagnosis, we include the deep learning technology into the diagnosis of burns.
Method
This article proposes a novel method employing a state-of-the-art deep learning technique to segment the burn wounds in the images. We designed this deep learning segmentation framework based on the Mask Regions with Convolutional Neural Network (Mask R-CNN). For training our framework, we labeled 1150 pictures with the format of the Common Objects in Context (COCO) data set and trained our model on 1000 pictures. In the evaluation, we compared the different backbone networks in our framework. These backbone networks are Residual Network-101 with Atrous Convolution in Feature Pyramid Network (R101FA), Residual Network-101 with Atrous Convolution (R101A), and InceptionV2-Residual Network with Atrous Convolution (IV2RA). Finally, we used the Dice coefficient (DC) value to assess the model accuracy.
Result
The R101FA backbone network gains the highest accuracy 84.51% in 150 pictures. Moreover, we chose different burn depth pictures to evaluate these three backbone networks. The R101FA backbone network gains the best segmentation effect in superficial, superficial thickness, and deep partial thickness. The R101A backbone network gains the best segmentation effect in full-thickness burn.
Conclusion
This deep learning framework shows excellent segmentation in burn wound and extremely robust in different burn wound depths. Moreover, this framework just needs a suitable burn wound image when analyzing the burn wound. It is more convenient and more suitable when using in clinics compared with the traditional methods. And it also contributes more to the calculation of total body surface area (TBSA) burned.
摘要:
Background
Burns are life-threatening with high morbidity and mortality. Reliable diagnosis supported by accurate burn area and depth assessment is critical to the success of the treatment decision and, in some cases, can save the patient’s life. Current techniques such as straight-ruler method, aseptic film trimming method, and digital camera photography method are not repeatable and comparable, which lead to a great difference in the judgment of burn wounds and impede the establishment of the same evaluation criteria. Hence, in order to semi-automate the burn diagnosis process, reduce the impact of human error, and improve the accuracy of burn diagnosis, we include the deep learning technology into the diagnosis of burns.
Method
This article proposes a novel method employing a state-of-the-art deep learning technique to segment the burn wounds in the images. We designed this deep learning segmentation framework based on the Mask Regions with Convolutional Neural Network (Mask R-CNN). For training our framework, we labeled 1150 pictures with the format of the Common Objects in Context (COCO) data set and trained our model on 1000 pictures. In the evaluation, we compared the different backbone networks in our framework. These backbone networks are Residual Network-101 with Atrous Convolution in Feature Pyramid Network (R101FA), Residual Network-101 with Atrous Convolution (R101A), and InceptionV2-Residual Network with Atrous Convolution (IV2RA). Finally, we used the Dice coefficient (DC) value to assess the model accuracy.
Result
The R101FA backbone network gains the highest accuracy 84.51% in 150 pictures. Moreover, we chose different burn depth pictures to evaluate these three backbone networks. The R101FA backbone network gains the best segmentation effect in superficial, superficial thickness, and deep partial thickness. The R101A backbone network gains the best segmentation effect in full-thickness burn.
Conclusion
This deep learning framework shows excellent segmentation in burn wound and extremely robust in different burn wound depths. Moreover, this framework just needs a suitable burn wound image when analyzing the burn wound. It is more convenient and more suitable when using in clinics compared with the traditional methods. And it also contributes more to the calculation of total body surface area (TBSA) burned.
阅读论文全文请访问:
https://burnstrauma.biomedcentral.com/articles/10.1186/s41038-018-0137-9?utm_source=other&utm_
medium=other&utm_content=null&utm_campaign=BSCN_2_WX_BurnsTrauma_Arti_Scinet
期刊介绍:
Burns & Trauma (https://burnstrauma.biomedcentral.com/) is an open access, peer-reviewed journal publishing the latest developments in basic, clinical and translational research related to burns and traumatic injuries. With a special focus on prevention efforts, clinical treatment and basic research in developing countries, the journal welcomes submissions in various aspects of biomaterials, tissue engineering, stem cells, critical care, immunobiology, skin transplantation, prevention and regeneration of burns and trauma injury.
(来源:科学网)
特别声明:本文转载仅仅是出于传播信息的需要,并不意味着代表本网站观点或证实其内容的真实性;如其他媒体、网站或个人从本网站转载使用,须保留本网站注明的“来源”,并自负版权等法律责任;作者如果不希望被转载或者联系转载稿费等事宜,请与我们接洽。